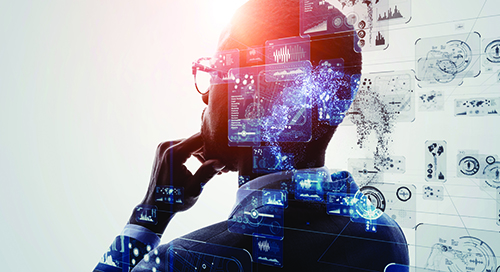
Artificial intelligence (AI) models in healthcare have the potential to improve the precision and speed of personalized medicine for patients, in some cases helping to identify the best treatment or preventive care. Clinicians are already implementing these models in areas such as early detection of sepsis and analyzing radiology images for diagnosis of prostate cancer and other conditions. It’s a growing area of interest that laboratory medicine professionals should pay attention to, as data generated by laboratory testing is a major component incorporated into AI tools to generate clinical decisions.
Clinical AI and a subset of AI known as machine learning (ML) can be used for tasks in a broad range of fields from precision medicine to population health, said Wade Schulz, MD, PhD, an assistant professor of laboratory medicine and a computational healthcare researcher at Yale School of Medicine in New Haven, Connecticut. The main advantage is speed because these tools rely on computerized instead of manual tasks. There’s a lot of interest in how to make AI/ML algorithms more vanced so they can predict more complex outcomes such as response to cancer treatment or risk for adverse events from surgery, he said.
Clinicians focus on understanding individual patients and their trajectories, explained Tylis Chang, MD, vice chair of pathology informatics for Northwell Health, chief medical information officer for the health system’s Pathology Service Line, and medical director for North Shore University Hospital Laboratories, in Manhasset, New York. But laboratorians have great skills in aggregating and processing data, said Chang, a member of AACC’s Data Analytics Steering Committee: “A natural extension of delivering a lab result is to deliver a risk analysis or a likely diagnosis. That’s really what all these predictive analytic tools are about.”
CURRENT AND POTENTIAL USES OF AI IN THE LAB
There has been some adoption of AI and ML techniques in the laboratory setting, primarily in molecular pathology (such as in classification of central nervous system tumors by DNA methylation profiling) and digital pathology (like image analysis), but it’s been going slowly, said Carlos J. Suarez, MD, associate director of the molecular pathology laboratory at Stanford University Medical Center in California and co-director of the Genetic and Genomic Testing Optimization Service. “It’s been there,” he said, “it just hasn’t been widely advertised.”
A review paper he coauthored (Clin Biochem 2022; doi:10.1016/j.clinbiochem.2022.02.011) highlighted some examples of AI/ML that have been studied in the lab, such as predicting laboratory test values, improving laboratory utilization, automating laboratory processes, promoting precision laboratory test interpretation, and improving laboratory medicine information systems—some with impressive accuracy.
For example, a study cited in the article used a neural network model to predict iron-deficiency anemia and serum iron levels based on features from a routine complete blood count. Another study cited discussed the development of a machine learning model capable of recommending what laboratory tests a provider should order. Overall, AI/ML technology holds promise to leverage large amounts of medical data to create more personalized interpretations of test results, the paper said, such that the paradigm could shift from defining a normal hemoglobin level in general to defining one for a particular individual.
Chemistry and immunology laboratories are particularly well-suited to leverage machine learning because they generate large, highly structured data sets, Schulz and others wrote in a separate review paper (Clin Chem 2021; doi: 10.1093/clinchem/hvab165). Labor-intensive processes used for interpretation and quality control of electrophoresis traces and mass spectra could benefit from automation as the technology improves, they said. Clinical chemistry laboratories also generate digital images—such as urine sediment analysis—that may be highly conducive to semiautomated analyses, given advances in computer vision, the paper noted.
Chang sees two overarching classes of AI models: those that tackle internal challenges in the lab, such as how to deliver more accurate results to clinicians; and those that seek to identify cohorts of patients and care processes to close quality gaps in health delivery systems.
The lab, however, “isn’t truly an island,” said Michelle Stoffel, MD, PhD, associate chief medical information officer for laboratory medicine and pathology at M Health Fairview and the University of Minnesota in Minneapolis. “When other healthcare professionals are working with electronic health records or other applications, there could be AI-driven tools, or algorithms used by an institution’s systems that may draw on laboratory data.”
HURDLES TO ROUTINE IMPLEMENTATION OF AI
Laboratories still must face significant challenges before the technology can be used in a more widespread manner. These include the need to collect high-quality data from diverse populations and to manage costs associated with computational infrastructure and personnel to develop and update algorithms and software tools.
In this relatively new field, there also have been no guidelines on best practices to clinically validate the algorithms. The College of American Pathologists within the past year formed a committee to help establish laboratory standards for AI applications. Although it’s still unclear what role federal and state regulators will take, the Food and Drug Administration has convened a committee to look into AI-driven devices, said Suarez.
Lack of familiarity with the technology also can be a barrier to selecting appropriate programs for use, said Suarez and Stoffel, noting that some people rely on the vendor’s marketing information, which can be overstated. In that case, laboratory professionals can ask for help from someone else at their institution knowledgeable about the field who can aid in assessing these models.
Understanding the different tasks that AI could assist with can help in approaching some of the challenges, Stoffel said. For people not as familiar with AI, trusting an AI model to do a diagnostic task that highly specialized staff or biologists are doing can present a big trust hurdle, Stoffel said, versus incorporating AI for something that seems lower risk, such as assessing workflow for areas that could be optimized, or detecting patterns in test utilization that could be improved. “These are things that would add value, and that nobody [in the lab] may have the bandwidth to do today,” she said.
Another approach is to implement an AI program alongside a manual process, assessing its performance along the way, as a means to ease into using the program. “I think one of the most impactful things that laboratorians can do today is to help make sure that the lab data that they’re generating is as robust as possible, because these AI tools rely on new training sets, and their performance is really only going to be as good as the training data sets they’re given,” Stoffel said.
There are ethical considerations when using AI in medicine, too. One is data access, and another is how to properly get consent from patients to include their data in larger pools, Schulz said. And, when the data is used, how can clinicians ensure they don’t introduce additional biases? “The models we produce are only as good as the data we collect,” Schulz said. “So if there are underlying biases in how we provide clinical care, those will potentially translate through to our models as well.”
AI models also may not help if the data set used doesn’t reflect the population served by a particular laboratory or health system, said Lucila Ohno-Machado, MD, PhD, MBA, the incoming deputy dean for biomedical informatics at Yale School of Medicine. Understanding and validating the models for individual settings is something important to do, she noted.
“Our job is to ensure that, whenever we do predictions for a particular patient that change the course of care, we are very convinced that the model is appropriate and it’s the best we can do at a particular point in time,” Ohno-Machado said, noting that models will need to be refreshed over time as new data and outcomes are collected.
BUILDING A BRIDGE TO THE FUTURE
In an effort to help with data access and related issues, the National Institutes of Health launched its Bridge2AI program to generate new flagship biomedical and behavioral data sets. The program also aims to define best practices for the collection and preparation of AI/ML data for biomedical and behavioral research. Ohno-Machado, Schulz, and some other laboratory experts are involved.
“It’s a very special program,” which aims to generate large amounts of data with the proper consent structure, “so those data can be used to produce AI models that introduce innovations in AI that can result in better health for individuals,” Ohno-Machado said.
“It’s a way of looking at it that is very different than the hypothesis-driven research that has traditionally been funded,” she added. “It’s funding to get large quantities of data in a very principled manner, and very standardized and organized so that AI can thrive from those large data collections.”
Schulz is involved in Bridge2AI projects focused on cell maps and integrating cell-level and clinical data into large databases. “It’s a very new area for clinical medicine,” he said.
In the future, AI will be incorporated directly into more devices and instruments, and laboratory directors might not be choosing standalone AI programs, Stoffel said. Instead, AI features may be incorporated into larger software packages they’re considering. “It’s going to allow us to move forward with many more precision medicine-based approaches and leverage a lot of biomedical knowledge that today does not make it into clinical AI and ML models,” Schulz said.
“I think it will be ubiquitous to the point that you don’t care if it’s AI or some other type of method to generate the results,” Ohno-Machado said. “With more and more data, the models tend to just get better. And the intention to have training data that is more diverse will mitigate some of the problems AI has had to date.”
Karen Blum is a freelance medical/science writer in Owings Mills, Maryland. +Email: [email protected]
Take a deeper dive into data in the January issue of The Journal of Applied Laboratory Medicine. The special issue, Data Science and the Clinical Laboratory, is available at academic.oup.com/jalm.